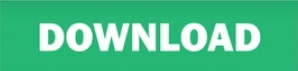
The team needs flexible and programmatic tools that can enable them to test ideas for AI projects quickly, iterating on those that are successful and customizing the tool for their unique use cases. Anna’s prime interest is Natural Language Processing but she also worked on audience segmentation and propensity modeling. In 2015, Anna decided to drastically change her career and become a data scientist. Previously she worked at the BBC where in 2007 she received an award for best producer in recognition of her success in building new audiences and developing an effective social media strategy. Anna leads a team of data scientists at the UK-based Guardian News and Media. About AnnaĪnna Vissens is a physicist by trade but has spent nearly all her career in media, first as a journalist, and then as a data scientist. Like Anna, the team has several members with backgrounds in natural sciences like physics along with many years of work experience in journalism. The Guardian data science team is a small team of six data scientists. Can you tell us about your team and what you’re working on? Along the way, they developed stakeholder trust through iterative group discussions yielding well-defined annotation guidelines and tandem team learnings during their annotation process. In a recent interview with Explosion, Anna Vissens, lead data scientist for the Guardian’s data science team, discussed how her team customized Prodigy to extract quote modules in news articles. For their AI projects, the Guardian’s data science team decided to use Prodigy, a modern annotation tool for creating training and evaluation data for human-in-the-loop machine learning.
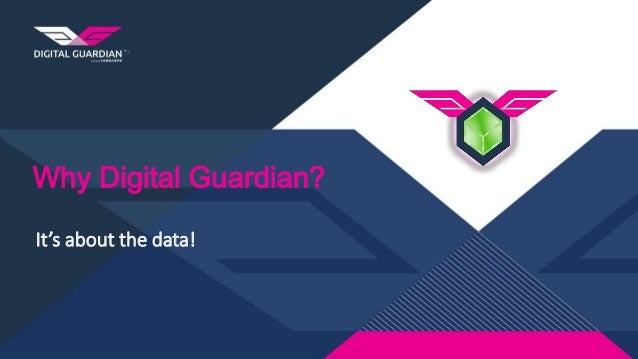
To facilitate trust, human-in-the-loop workflows are widespread in media applications as stakeholders require the ability to teach and to evaluate models through human-AI interfaces. Anna Vissens, lead data scientist for the Guardian. We’re very much aware of potential reputational risks… in media trust is everything… very easy to lose and hard to build up again. Therefore, model stakeholders like journalists and editors must trust and review AI models before deploying them for customer-facing content generation. Given audiences of potentially millions of global viewers, one wrong automation can lead to many dissatisfied news consumers. The Guardian explored quote extraction with the goal of modular journalism to reuse quotes from long articles for different media artifacts like podcasts or information graphics.Īt the same time, a push towards automating reusable content may incur significant reputational risks for media companies. This push is called modular journalism and many media companies are building towards it to automate customized stories to meet individual user needs for a variety of media forms. In the episode, Daniel mentions: Darren Wickham, Francis Burgess and Andrew Yin.A recent trend for media companies is to explore how fields like Natural Language Processing (NLP) and Information Extraction (IE) can modularize content like a long-form article as reusable elements for different storytelling formats (e.g., a podcast, information graphic, or blog). His top tip for actuaries wanting to improve their skills in data science. How he sees the competitive landscape between actuaries, data scientists and other data analytics professionals, and What skills he looks for when hiring in his teams, How he balances the need to deliver without overloading team members, His advice to experienced actuaries that are wondering if it’s “too late” to learn data science and apply it to their roles, How he became on of the first life insurance actuaries in Australia who adopted data science into their roles, In his spare time he enjoys spending time at the beach with his family and making a deep run in poker tournaments. His recent experience is in applying big data and cloud technologies, data engineering automation and the application of statistical and data science techniques in life insurance and actuarial pricing. He has worked in Australia, South Africa, United Kingdom and ASEAN. Daniel Stone is a qualified actuary and data scientist with 20 years of experience in a wide range of fields including varied-sized life offices, reinsurance, consulting and expert-level model building experience.
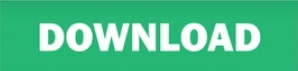